Content marketing and data
Many of the marketing directors and managers we speak to and work with accept that content marketing and social media are important. Some of the reasons include the following:
- Getting traction on social media to win more followers
- Increasing the site traffic levels by increasing referral sources
- Earning links from sites that have real audiences and traffic
- Increased brand awareness
However, getting content and social media marketing campaigns running invites many questions ranging from the planning to the operational including:
- How beneficial will the content marketing be for securing budget?
- Which topics should we write about?
- Which influencers should we approach?
- How do we calculate and report the ROI?
While no single source of data could ever hope to be the basis upon which to answer all those questions, BuzzSumo in our opinion is the best commercially viable data source for content marketing and social media on the market today, at the this time of writing.
Data without analysis of course will yield very little useful insight that marketers can use to make their campaigns successful.
Enter the machine
Machine learning is a branch of artificial intelligence that allows us to spot patterns at scale. While humans are very good at spotting patterns in general, there is always a risk that humans will find patterns that don’t actually exist. Machine learning on the other hand, uses statistics to validate patterns. Before machine learning can be deployed, we need the data.
Most, if not all, data scientists would agree that getting the data in is 95% of all data science work, (the percentages among data scientists may vary). Thankfully Buzzsumo offer an API which gives us the ability to ‘pipe’ their data into our technology platform to start doing some machine learning. Their API guide is immensely available which is available here. For those of you not conversant with APIs, the BuzzSumo interface has a CSV download which can still be analysed using machine learning using packages like MATLAB and R.
So for our particular brief we were asked by one of the UK’s leading online estate agents to understand why some content marketing in their industry performs better than others whether it’s content produced by their competitors or content about the issues that are industry relevant such as ‘landlords rights’ for example.
Using BuzzSumo, we put together a dataset covering 30,000 URLs that comprised searches on:
- websites in the online estate agent industry in the UK
- search phrases containing topical phrases such as ‘landlord rights’, ‘estate agents etc
With the dataset, we deployed machine learning techniques in order to see if we could find any patterns that would help us guide the content marketing strategy. In this particular exercise, the following are worth noting:
- we are trying to predict content with a high amount of social media shares
- we are trying to identify ‘features’ (i.e. predictors) that lead to higher shares on social media
With the above in mind, we will train a portion of the dataset before testing the model on an ‘unseen’ portion of the dataset to test and refine the accuracy of the model. The process will be repeated until the model can no longer be improved without gathering more data.
The results
After several iterations, the machine learning exercise produced a model with a low error rate:
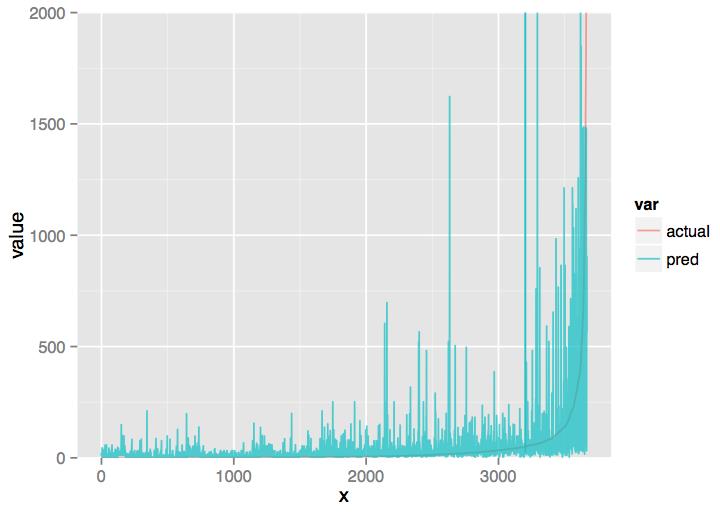
Graphic: Error rates
We can see that the model fits particularly badly for posts with high number of shares, as might be expected. The median error is around 10.14 which means that on average the model predicts a number of shares a content piece will get is wrong by about 10. However, some of the time the model is closer than this. Other times, particularly for a pages with a high number of shares the model is less accurate.
So what are the predictors that our model picked out?
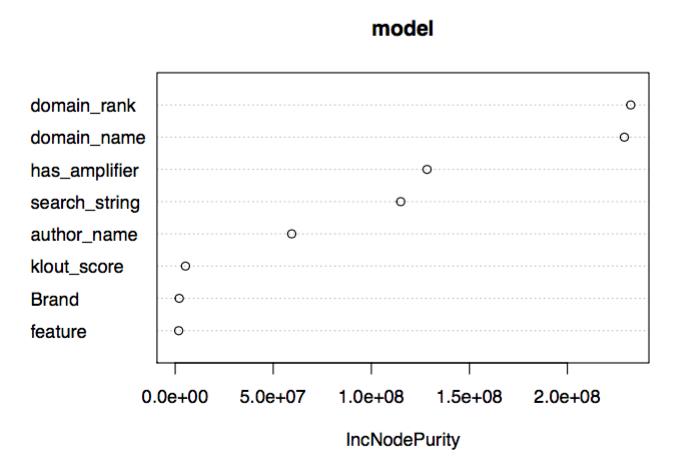
Graphic: Predictors
Looking at the above chart shows that the strongest predictors are:
- domain rank (an estimated measure of a site’s PageRank or domain authority)
- domain name
- amplifier (the number of times an article got retweeted)
- topic (the search string used to query BuzzSumo)
- author
All of the above predictors with the exception of ‘domain rank’ are available from the BuzzSumo data feed. Surprisingly, the Klout score was not as predictive in helping us predict content for the online estate agency industry.
Without giving away client ‘paid for’ confidential insights away, machine learning has helped the content strategists identify patterns with their associated benchmarks that they should aim for when putting together a content marketing strategy that is highly likely to succeed. Below are some takeaways that content strategists would now know as a result of machine learning:
- the minimum domain authority of bloggers to engage with (domain rank)
- the existing influencer sites (domain name)
- which people on social media will be worthwhile amplifiers
- the number of amplifiers per article are required
- which topics get traction
- which authors perform well and may be considered true influencers
Unanswered questions
There are still unanswered questions as to other un-modeled drivers behind successful social media campaigns. The one that come to our mind are:
- How does the general news trends affect the social traction of content?
- Does it matter if content gets shared on Facebook (or any other channel) first?
Concluding thoughts
Whilst machine learning may confirm what an expert content strategist might already know, some of the insights will be surprising and most of the insights will have numerical benchmarks. Machine can’t explain everything and the model is only as good as the accuracy of the data and the number of data fields from which the algorithm has to draw from.
Computers are still rather bad at reading and understanding text also, despite the advances in text mining and neuro linguistic processing (NLP).
Despite the challenges and limitations of data science for making content marketing more predictable, we should nevertheless use data sources like BuzzSumo and deploy machine learning to extract insights and make content marketing more data driven. The advances in computing, the availability of data and the demands of our colleagues and clients compel us to do so.